DATA MERGING AND GIS INTEGRATION IN DIGITAL IMAGE PROCESSING
Learn about data merging and GIS integration techniques in digital image processing, including their applications and limitations.
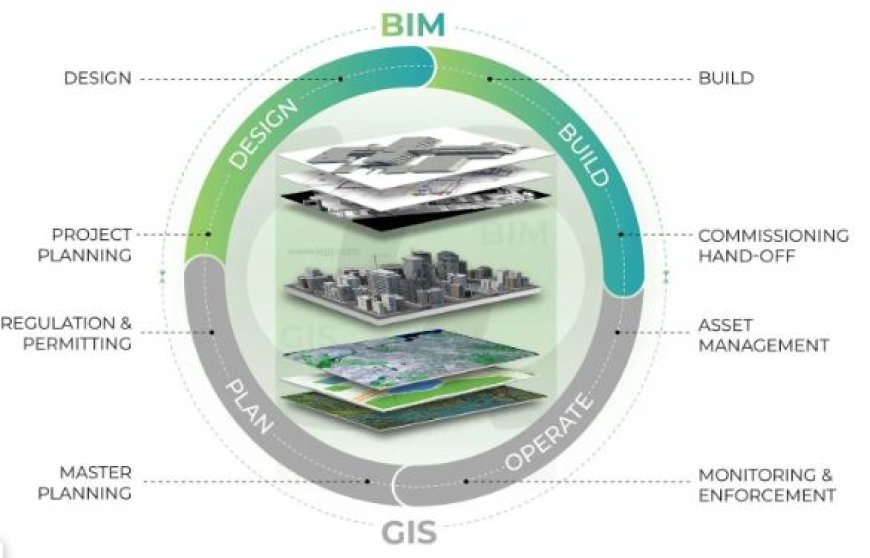
Data Merging and GIS Integration in Digital Image Processing
- The use of remote sensing has fundamentally transformed the methods by which we gather and scrutinize data pertaining to the Earth's surface.
- Remote sensing technologies provide extensive data that need advanced techniques for analysis and interpretation, serving many fields such as environmental monitoring and urban planning.
- An essential method in remote sensing is the integration of several data sources, typically aided by Geographic Information Systems (GIS).
Understanding Data Merging in Remote Sensing
- Data merging in remote sensing refers to the act of integrating numerous datasets to generate a more comprehensive image of an area of interest.
- This process may entail the fusion of pictures obtained from various sensors, satellites, or aerial platforms, along with the incorporation of other information, such as vector data depicting highways, land use, or hydrology.
- The use of various sensors allows for the capture of distinct spectral bands and spatial resolutions, which in turn enables the identification of different features on the Earth's surface.
- By combining these datasets, we may acquire more comprehensive information, which will enhance the accuracy of feature identification and categorization.
- Enhanced Precision: Integrating data from multiple sources can result in heightened accuracy, particularly when addressing atmospheric correction or sensor errors.
- Incorporating supplementary data can enhance the accuracy of the outcomes derived from remote sensing pictures.
- Comprehensive Analysis: Various aspects of analysis, such as detecting changes in land use, fragmentation of habitats, and managing disasters, necessitate data from multiple sources.
- By merging data, researchers and analysts may do more comprehensive investigations.
The Role Of Geographic Information Systems (GIS) In Data Integration
- Geographic Information Systems (GIS) are essential for the integration of diverse datasets.
- GIS offers the necessary tools to efficiently handle, examine, and depict geographical data.
It functions as the key support for combining data by enabling users to:
- Manage and Arrange Varied Data: GIS has the capability to process raster (picture data) and vector (point, line, and polygon data) formats, allowing users to quickly arrange different types of information.
- Geographical analysis refers to the use of GIS to conduct complex analyses of geographical relationships between various datasets.
- This includes doing overlay analysis and generating new datasets based on the results.
Visualization: Geographic Information System (GIS) technologies provide the representation of combined datasets on interactive maps, simplifying the comprehension of intricate data and facilitating the transmission of findings to interested parties.
Techniques for combining and integrating data with Geographic Information Systems (GIS)
- Data merging and GIS integration involve several techniques, each with its own distinct uses:
1. Resampling
- When combining photos from various origins, it could be essential to resample the data to a uniform pixel size.
- Methods such as bilinear interpolation or closest neighbor resampling can be employed to modify the spatial resolution of the pictures being combined.
2. Image Registration
- Ensuring that multiple datasets align correctly is crucial in data merging.
- Image registration is the process of matching pictures captured by separate sensors or taken at different times to a shared coordinate system. This is often achieved by employing ground control points (GCPs) to guarantee precise alignment.
3. Analysis using overlay techniques
- After aligning datasets, it is possible to do overlay analysis using GIS. This process entails integrating data from many levels, such as superimposing satellite images with land use data, in order to detect patterns and correlations.
- Principal Component Analysis (PCA) is a statistical technique used to reduce the dimensionality of a dataset while retaining as much information as possible.
- It is commonly used in data analysis and machine learning to identify the most important features or variables that contribute to the overall variance in the data.
- PCA is a technique that may be employed to combine data, aiding in the reduction of complexity while preserving important details.
- PCA helps improve feature extraction in combined datasets by converting correlated variables into a set of uncorrelated values.
Applications of Merged Data in Remote Sensing
- Data merging and GIS integration have extensive applications in the field of remote sensing, including a diverse array of uses.
- Environmental monitoring involves the identification of alterations in land cover, the examination of deforestation rates, and the evaluation of the impacts of climate change on ecosystems.
- Urban Planning: Facilitating land-use planning, infrastructure development, and monitoring urban sprawl by combining demographic and satellite data analysis.
- Disaster Management: Enhancing reaction times and resource allocation by utilizing integrated datasets for real-time evaluations during natural catastrophes.
- By integrating satellite data with crop yield data, farmers may engage in precision agriculture, allowing them to make informed decisions based on data to optimize production.
IMAGE SOURCE (THUMBNAIL)
What's Your Reaction?
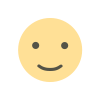
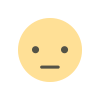
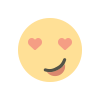
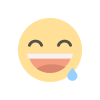
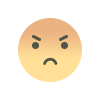
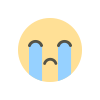
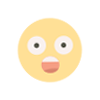